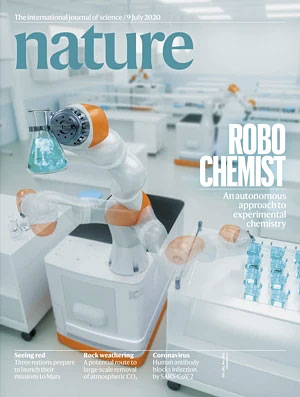
The discovery of new materials is a time-consuming and labor-intensive task, just as Edison tested thousands of filament materials during the invention of the light bulb. The traditional trial and error method has extremely high costs when searching for new materials, which seriously restricts the rapid discovery of new materials. However, in recent years, automated exploration models that integrate robotics technology and active learning strategies have emerged, thanks to the breakthrough progress of artificial intelligence (AI) combined with robotics technology and deep learning in multiple fields, playing a key role in materials science research.
The research team of Google DeepMind has published a heavyweight article titled "Scaling deep learning for materials discovery" in Nature (Nature, 2023, 624, 80), which explores in depth how to significantly accelerate the discovery process of new materials using deep learning technology. The technology they used is called "Graph Networks for Materials Exploration (GNoME)", which has successfully predicted the structures of 2.2 million new materials, many of which do not conform to the chemical "intuition" of human scientists. Over 700 of these structures have been successfully synthesized in the laboratory.
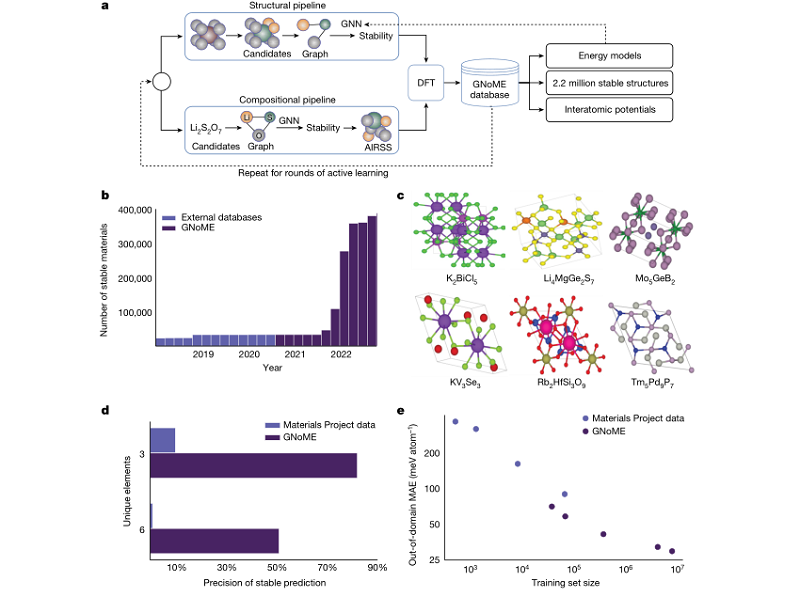
Predicting the existence of materials is one thing, but actually manufacturing them and verifying their potential applications is another challenge. In traditional manual laboratory operations, developing new materials is a very time-consuming process. Researchers at Lawrence Berkeley National Laboratory (LBL) have developed an automated laboratory platform called A-Lab (Nature, 2023624, 86) aimed at shortening the time gap from computational screening to experimental synthesis of new materials. A-Lab utilizes computational models, historical literature data, machine learning, and active learning techniques, combined with robot automation, to optimize the design and synthesis process of new materials.
The A-Lab platform utilizes materials discovered from GNoME and Materials Project data, and through machine learning model training, is able to autonomously determine the optimal method for synthesizing target materials and create combinations of up to five initial raw materials. After each experiment, A-Lab will adjust the raw material combination based on the experimental results. In just 17 days, A-Lab completed 355 experiments and successfully synthesized 41 out of 58 compounds, including various oxides and phosphates. Even cases of failed synthesis provide valuable feedback and suggestions for improving material screening and synthesis design techniques, which have direct and practical application value for optimizing existing technologies.
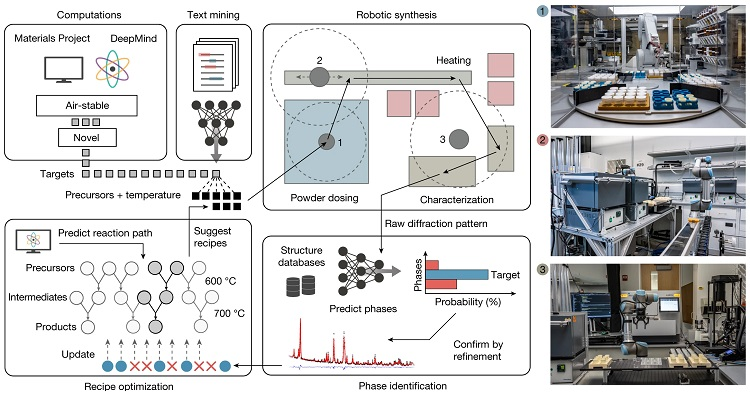
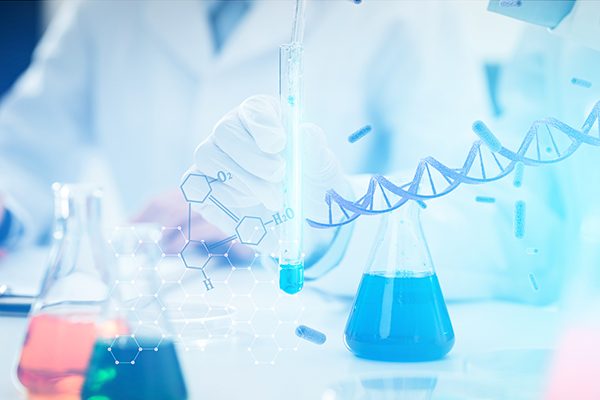
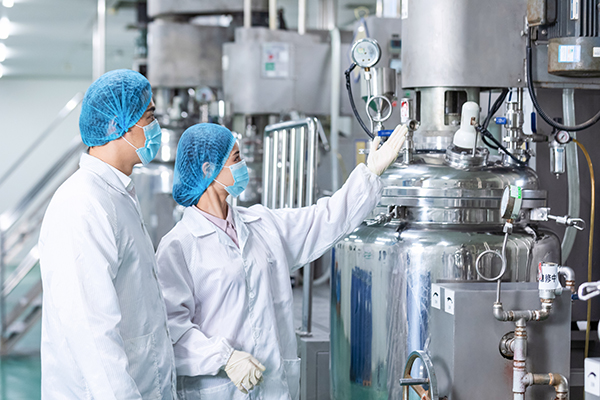
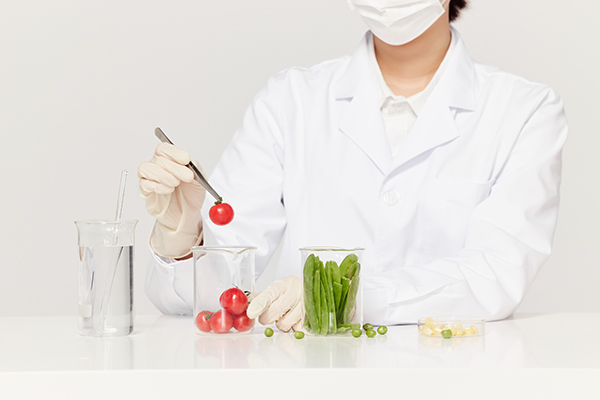
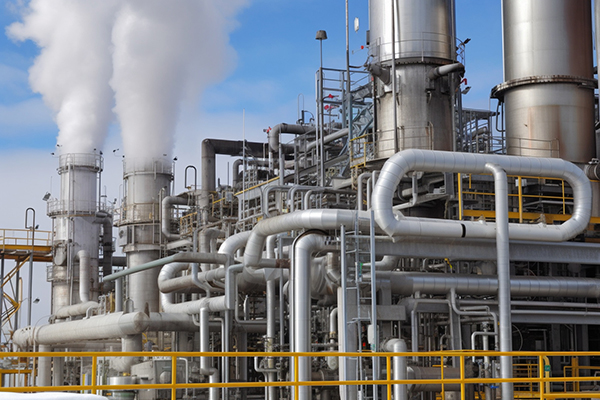